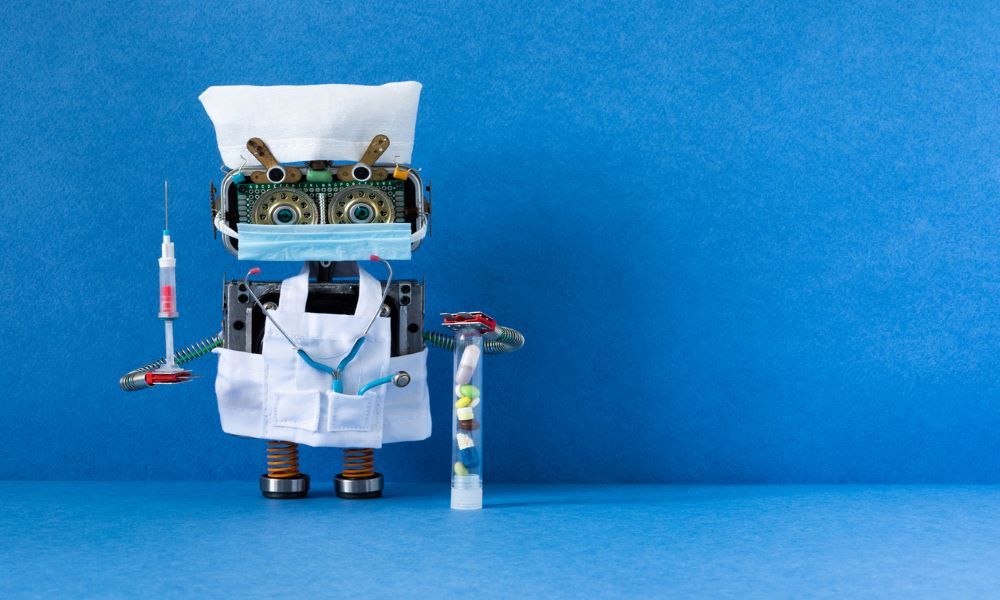
The spectre of Ferguson lives on
In a sane world, the legitimacy of using computer modelling as a rationale for imposing covid restrictions would be seriously questioned. In 2020, Professor Neil Ferguson (an epidemiologist at Imperial College London) gained notoriety for his doomsday prediction that 2.2 million Americans and 500,000 British people could die as a direct consequence of the novel SARS-CoV-2 virus. These wildly inaccurate prophesies were largely responsible for spooking governments into imposing ineffective and hugely damaging lockdowns and, subsequently, the modelling methodology was heavily criticised. Yet it has recently been revealed that NHS England are relying on this flawed approach to justify their mask requirements in healthcare settings.
In response to a letter from the campaign group Smile Free criticising the persistence of mask wearing in healthcare settings, NHS England revealed that their policies on the use of face coverings are continuing to be shaped by the woeful predictions emanating from computer modelling. Replying on behalf of Amanda Pritchard (NHS England chief executive), Dame Ruth May (the national lead for infection control) repeated the often-heard claim that universal masking in hospitals would significantly reduce viral transmission. In support of this assertion, Dame May references an October 2021 pre-print study from the UK Health Security Agency (UKHSA) that describes how modelling had concluded that, if everyone wore a mask, SARS-CoV-2 infections among healthcare staff would reduce by 46%. In addition to a failure to recognise the robust real-world evidence aggregating around the conclusions that masks are both ineffective and harmful, it is astounding that NHS England are relying so heavily on this unreliable methodology.
This attempt by NHS England to reference modelling in order to justify the persistence of their mask requirements can be criticised in several ways. In general terms, this type of study harbours several inherent flaws, including: wrong assumptions about the modelling rules (such as the premise that everybody is at equal risk of infection); lack of transparency around the methodology leading to coding errors; groupthink where modellers fit their prediction to what is the dominant narrative and expectation; and inaccurate assumptions about key features of the pandemic. Arguably, the latter flaw is often the most consequential. Whereas Ferguson’s 2020 prophesies of Armageddon were significantly influenced by his overestimation (by at least four-fold) of the SARS-CoV-2 infection fatality rate, the UKHSA study cited by NHS England dubiously assumes that masks achieve a significant reduction in viral transmission.
Contrary to the findings of most of the rigorous, in-vivo studies, the authors of the UKHSA article cling to the premise that masks are effective in curbing the spread of infection and include this presumption in their modelling. This was despite their prior acknowledgements of there being, ‘Important gaps in the evidence base’ and that, ‘Evidence around the efficacy of interventions such as wearing surgical masks … is severely lacking’. So assume masks work and – surprise, surprise – the model concludes that this intervention reduces infections in healthcare staff. It is astounding that NHS England are relying on such circular logic to defend their controversial mask policies.
The pre-print status of the UKHSA study also screams that its findings fall well short of an evidential bar that would justify the imposition of masks in our hospitals. The lack of peer review, and the explicit cautionary note at the start of the article that, ‘it should not be considered conclusive, used to inform clinical practice or referenced by the media as validated information’ further highlight the absurdity of NHS England using it to justify their mask policies.
Once again, it appears that – as far as state-imposed covid restrictions are concerned – ideology and culture are trumping rationality.